Multiple UDFs
UDF work best when handling small amounts of data at time, but often datasets are large. This is where running UDFs in bulk becomes needed
There are 2 main approaches to running multiple UDFs:
- Using "offline" instances
- For high latency, large volumes of data
- Using "real-time" instances
- For small requests to finish quick
A Simple UDF
Here's a simple UDF:
@fused.udf
def udf(val):
import pandas as pd
return pd.DataFrame({'val':[val]})
As mentioned in the Single UDF section, to call it 1 time we can do:
fused.run(udf, val=1)
Which returns:
| val
------
0 | 1
We then have 2 options to run this multiple times:
Using "offline" instances (run_remote()
)
When to use: This is for high-latency (anything more than a few seconds) parallel processing of longer or more complex jobs
Running "offline" job
Using the same UDF as above we can run this UDF 10 times on an "offline" instance:
# We'll run this UDF 10 times
job = udf(arg_list=range(10))
job.run_remote()
This pushes a job onto a persistent machine on Fused server.
run_remote
additional arguments
With job.run_remote()
you also have access to a few other arguments to make your remote job fit your need:
instance_type
: Decide which type of machine to run your job on (based on the AWS General Purpose instance types)disk_size_gb
: The amount of disk space in Gb your instance requires
For example if you want a job with 16 vCPUs, 64Gb of RAM and 100Gb of storage you can call:
job.run_remote(instance_type="m5.4xlarge", disk_size_gb=100)
Accessing "offline" job logs
You can view the logs of all your on-going and past run_remote()
jobs either:
In a notebook
Running job.run_remote()
in a notebook gives you a clickable link:
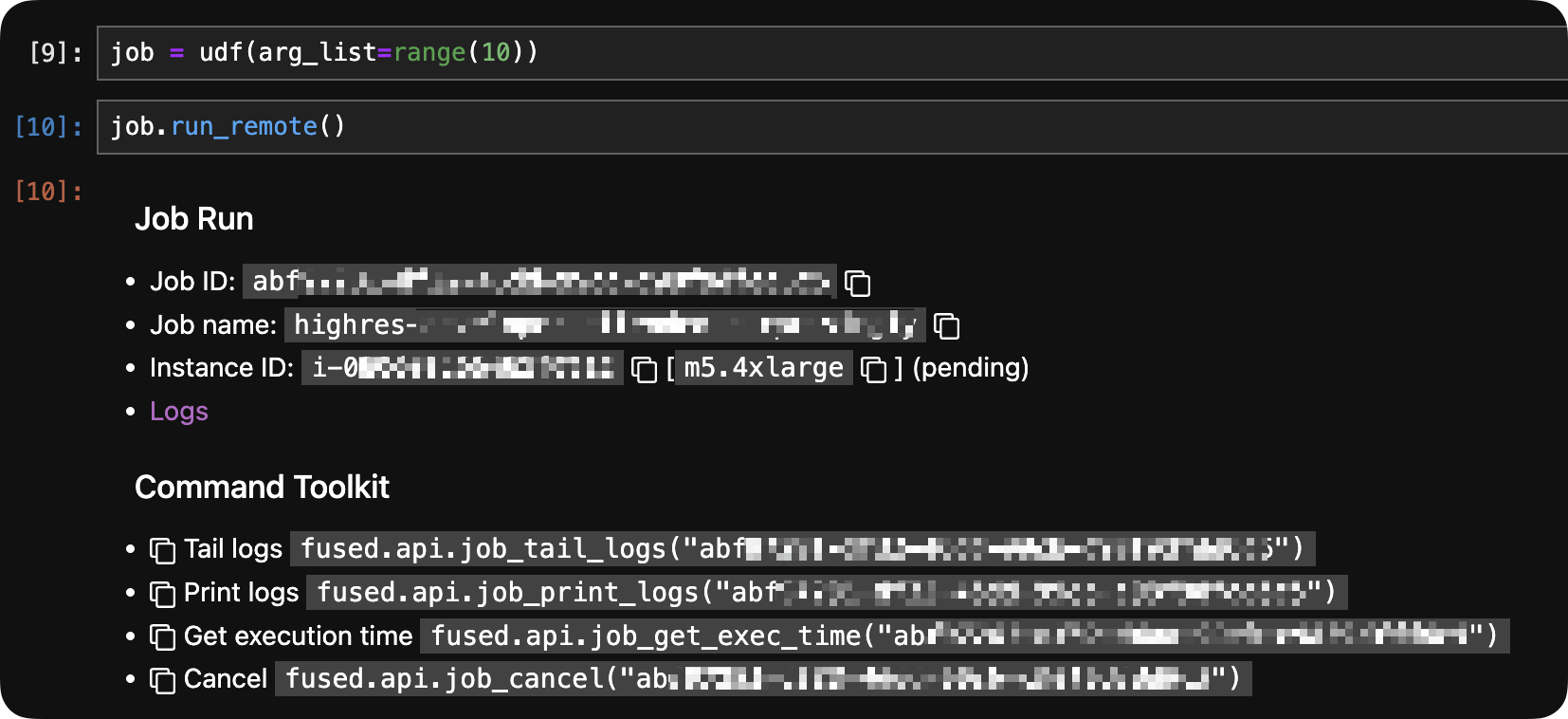
In Fused Workbench
Under the "Jobs" tab, on the bottom left of Workbench:
By email (you'll receive 1 email for each job)
Each job leads to an email summary with logs upon completion:
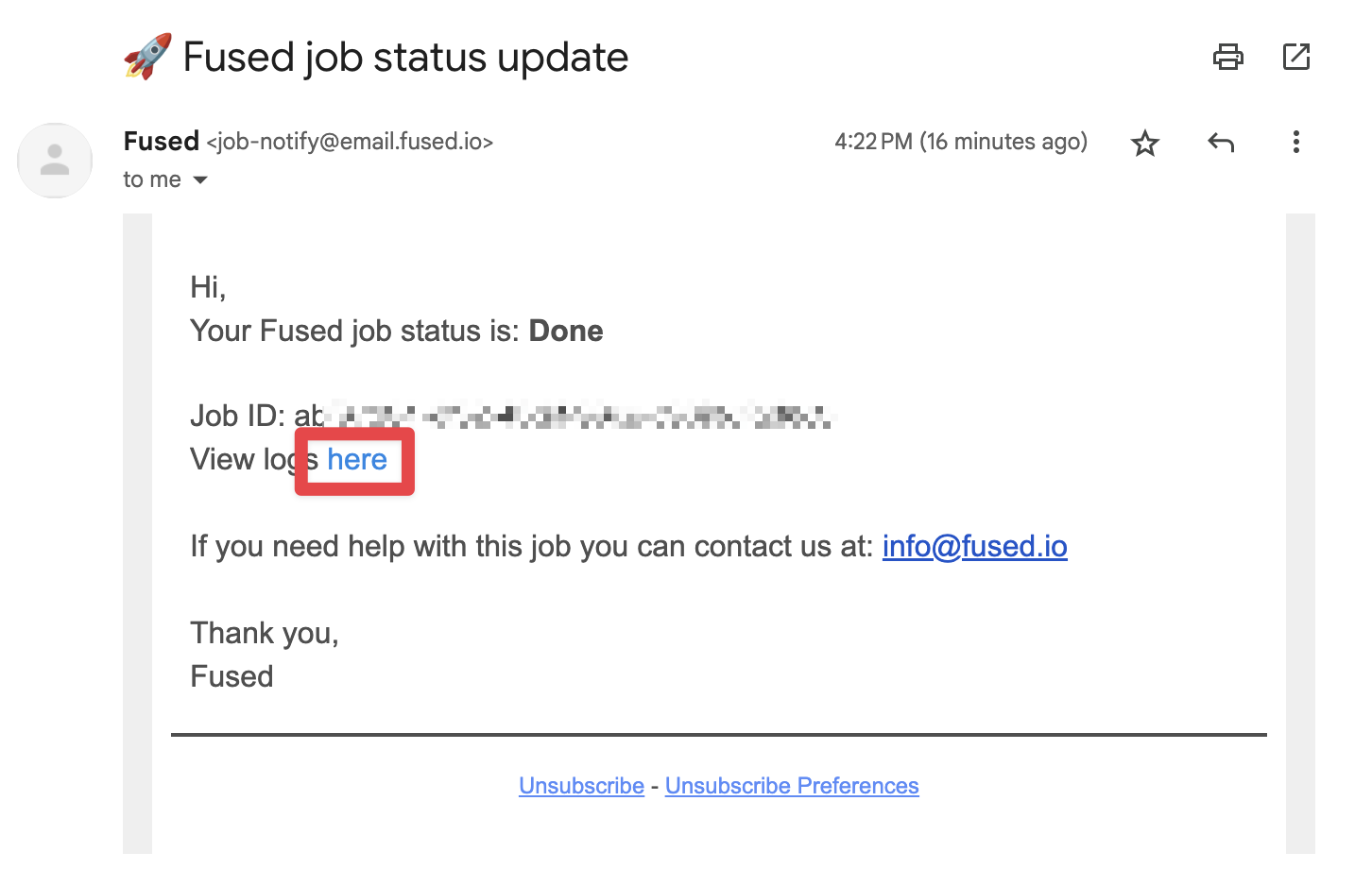
Getting "offline" results
To get data back from your "offline" run is a bit more complicated than for "real-time". Our recommendation is to have your UDF write data directly to disk or cloud storage and access it after
Example job: saving to disk
A common use case for offline jobs is as a "pre-ingestion" process. You can find a real-life example of this in our dark vessel detection example
Here all we're returning is a status information in a pandas dataframe, but the our data in unzipped, read and saved to S3:
import fused
@fused.udf()
def read_ais_from_noaa_udf(datestr='2023_03_29'):
import os
import requests
import io
import zipfile
import pandas as pd
url=f'https://coast.noaa.gov/htdata/CMSP/AISDataHandler/{datestr[:4]}/AIS_{datestr}.zip'
# This is our local mount file path,
path=fused.file_path(f'/AIS/{datestr[:7]}/')
daily_ais_parquet = f'{path}/{datestr[-2:]}.parquet'
# Download ZIP file to mounted disk
r=requests.get(url)
if r.status_code == 200:
with zipfile.ZipFile(io.BytesIO(r.content), 'r') as z:
with z.open(f'AIS_{datestr}.csv') as f:
df = pd.read_csv(f)
df.to_parquet(daily_ais_parquet)
return pd.DataFrame({'status':['Done']})
else:
return pd.DataFrame({'status':[f'read_error_{r.status_code}']})
Since our data is written to cloud storage, it can now be accessed anywhere else, through another UDF or any other application with access to cloud storage.
Tradeoffs:
- Takes a few seconds to startup machine
- Can run as long as needed
[Experimental] Using "real-time" instances (run_pool
& PoolRunner
)
When to use: This is for quick jobs that can finish in less than 120s
This is not a feature directly implemented in fused
. Instead we're going to use a code from the fused.public.common
utils module, that might change over time
You can learn more about import utils from UDFs here
If you want to quickly run a UDF a few times over a dataset, you can use PoolRunner
. Using a lambda
function you can map the function to pass to PoolRunner
:
runner = fused.utils.common.PoolRunner(lambda val: fused.run(udf, val=val), range(10))
runner.get_result_all()
In a notebook runner.get_result_all()
prints the progress status over time until the job is done:
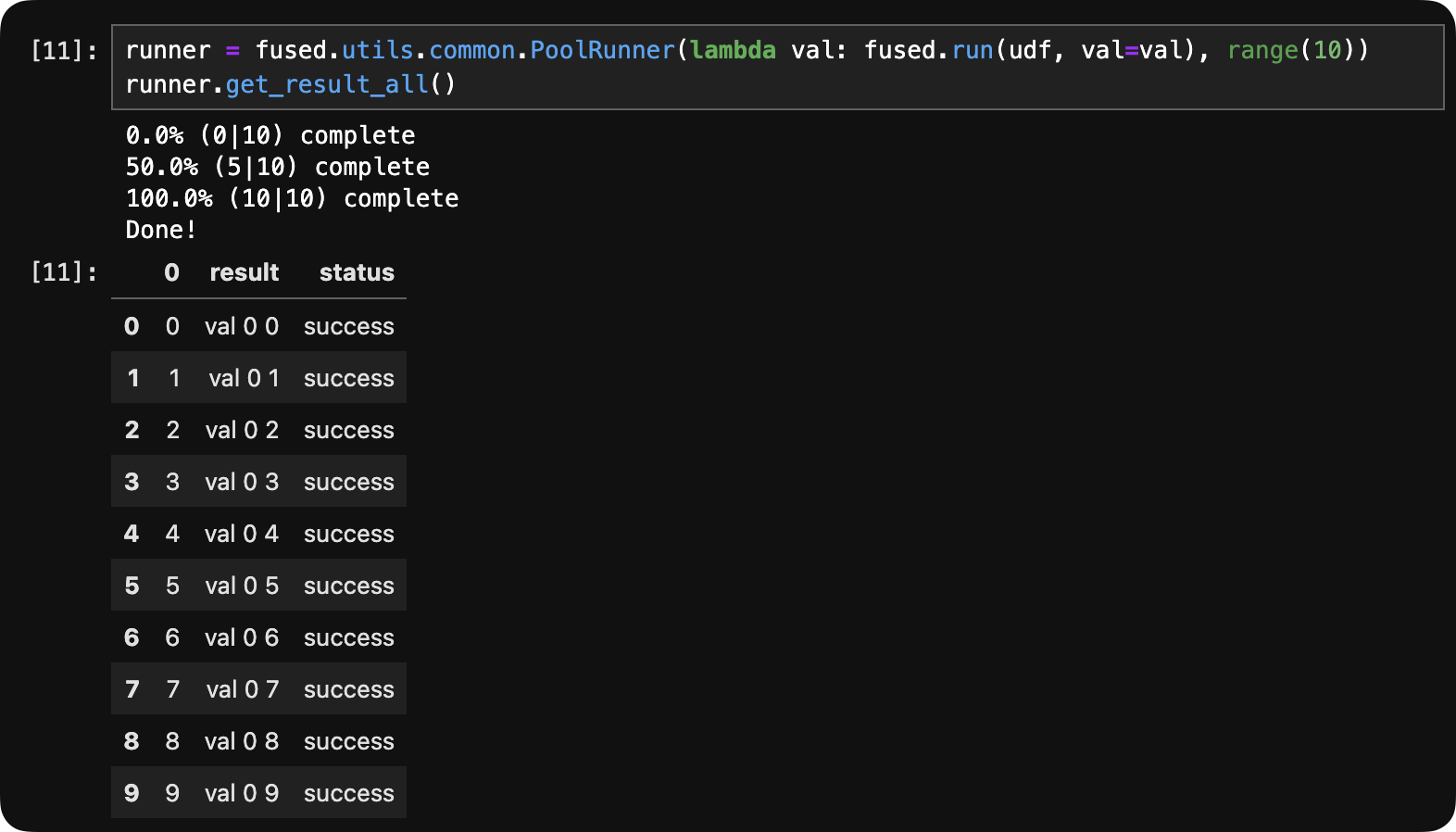
Getting "real-time" results
You can then get all your results by concatinating them:
# In this example udf() returns a Pandas DataFrame so `.get_concat()`
result = runner.get_concat()
with type(output)
being a pandas.core.frame.DataFrame
Tradeoffs:
- No startup time
- Will timeout after 120s